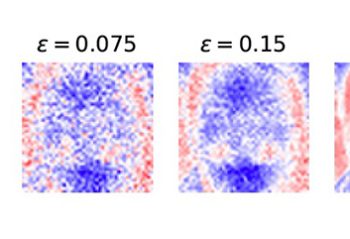
Full Abstract: Black-box machine learning models are used in critical decision-making domains, giving rise to several calls for more algorithmic transparency. The drawback is that model explanations can leak information about the data used to generate them, thus undermining data privacy. To address this issue, we propose differentially private algorithms to construct feature-based model explanations. … Continue reading "Model Explanations with Differential Privacy"
Read More