Towards an AI Accountability Policy
By
It’s a new day for AI. AI systems can assist us in writing a new web script, decide whether we should worry about that weird spot in an X-ray, and find friends on social media. AI systems help in determining recidivism risk. Music-generating AI can render novel songs by Drake and the Weekend — that were never sung by them. The software we’re using to write this blog post has a little widget enthusiastically suggesting better ways of shortening, and formalizing our paragraphs. Indeed, text generating AI systems are transforming the education, legal, and marketing ecosystems almost overnight. AI can also create realistic videos of politicians saying or doing controversial things, flood your social media feed with biased information, write misleading essays, and offer suggestions that disadvantage women and minorities.
AI’s benefits and risks are a rapidly evolving, disruptive force in the modern world. In light of this, governments, corporations, and individual customers should ensure that they are safe and align with societal and legal norms. We should also be aware of AI’s limitations, and (as AI experts) be extremely careful of overstating its capabilities. AI systems often reflect the values of their developers and vary in terms of their political, corporate, and national views. This raises the question of how governments can incentivize AI system designs that best fulfill the needs, interests, and safety of various stakeholders.
We recently submitted a white paper that offers interconnected recommendations for an AI accountability policy in response to the NTIA’s Request for Comments. AI accountability regulations and practices can make a significant difference even if legal standards and enforceable risk thresholds are introduced solely for the purpose of maintaining transparency among the AI system applications, rather than gatekeeping AI systems development. A public AI registry that certifies and gathers information about AI systems would motivate AI developers to compete in the area of AI accountability; this is similar in spirit to vehicle safety standards, which in turn encourage car manufacturers to offer better safety features. We anticipate that comparing AI systems on objective and transparent grounds will positively impact their design.
We believe in lightweight regulation. Overregulation (e.g. mandatory licensing to develop AI technologies) could frustrate the development of trustworthy AI, since it would primarily inhibit smaller actors from participating in AI development. The white paper adapts ideas from the European Union’s AI Act and prior work that calls for a new federal office to regulate facial recognition AI systems, while offering ways to foster competition in the AI ecosystem. We do not presume to offer a precise structuring of such an office within the federal government; we do, however, discuss the necessary elements to establish sorely needed controls for AI systems.
From Open-world Benchmarks to AI Certificates and Registry
OpenAI released a widely circulated report evaluating its flagship and popular GPT-4 model. This report suggests that GPT-4 performs exceptionally well on various tests, ranging from professional licensing exams to coding competitions. Unfortunately, later it turned out that these performance scores are likely overestimated due to so-called data contamination (see details in this blog post by Arvind Narayanan and Sayash Kapoor). This problem is more general and known in AI research as model overfitting or training data memorization and results in lower performance on deployment of AI systems in the real world. In other words, while AI systems do really well on data of the sort that they have previously observed, they tend to do really badly (or do really weird things) when faced with completely new data.
In our white paper, we propose the development of more diverse and realistic benchmarks for testing AI systems within different deployment types. We argue that these benchmarks shall maintain a barrier between the evaluated AI systems and the entities that evaluate them, following the evaluation structure developed by DARPA’s SAIL-ON program. The barrier separates AI system manufacturers and auditors, which enables testing AI systems on novel input data, hence avoiding the data contamination problem. The SAIL-ON evaluations of multiple AI agents in multiple environments show that contemporary AI is far from achieving robust, flexible, and adaptive performance on a human level. Significantly more research and development focused on robustness is needed to achieve such capabilities.
To incentivize such research and development, and to improve assurances that AI systems are ready for deployment, we argue for automated benchmarking audits. The results of such audits and benchmarks would need to be clearly and transparently communicated, explained, and compared across competing AI systems, and could lead to standard certificates for AI systems. These audits, benchmarks, and certificates shall be specific to deployment type categorized by risk levels. We argue that only AI systems applied in high-risk deployment areas should be fully registered, described, and audited (see Figure 1).
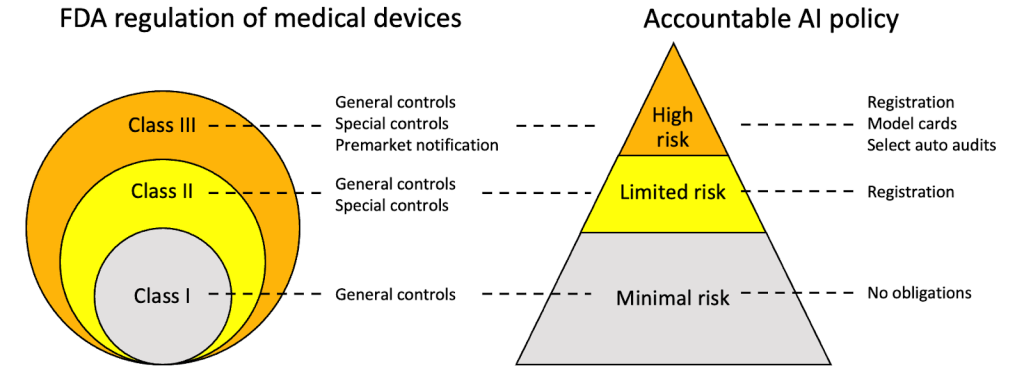
Fairness via Explanations
The importance of AI system comparisons and explanations is particularly evident in the employment sector. Comparisons and explanations of employment practices play a central role in the burden shifting framework used in the U.S. courts to determine employment discrimination. This framework relies on explanations and comparisons of employment practices. To prove discrimination, one must point to an “alternative employment practice” that alleviates disparate impact while achieving the business necessity. Proving that such an alternative exists is difficult, since typically plaintiffs and legal clerks lack information about the alternatives.
To address this issue and integrate AI systems with the burden shifting framework, AI accountability mechanisms shall enable comparisons among AI systems. We suggest using explainable AI techniques, such as input influence measures, to explain AI systems. For example, such input influence measures cand identify discriminatory credit rating models that use a protected attribute (such as race, see Figure 2A) or its proxies (such as zip code, see Figure 2B). In recent work, described in this blog post, we use such input influence measures to develop non-discriminatory AI systems. The technique inhibits direct discrimination in historical training datasets and enables a restricted use of business necessity attributes that correlate with protected attributes, by preventing their use as proxies for the protected attributes (Figure 2C). Now that the Supreme Court of the U.S. ruled against affirmative actions (Figure 2D), the burden shifting framework and approaches that align with it will likely grow in importance, e.g., the framework was recently proposed in the housing sector.
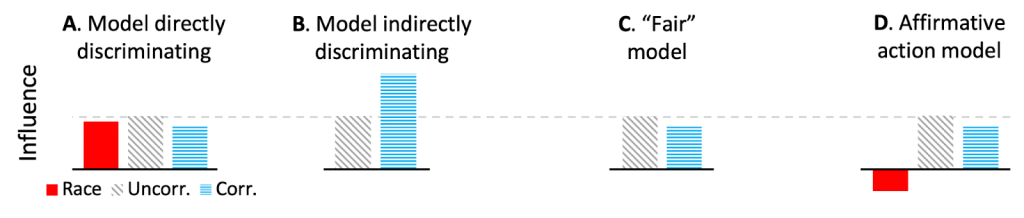
Social Media Transparency
Social media does not sufficiently inform its users about the provenance and authenticity of content spreading on its platforms, even if that content has political relevance. For instance, deep fakes generated by AI systems can spread and AI bots can respond to political polls on social media. While social media do not have ground truth information about the provenance of content and user responses, such platforms widely use AI systems to identify provenance of content. For instance, Twitter approximately from 2016 to 2023 identified the inauthentic votes in Twitter polls that were purchased on external websites, but displayed them publicly as authentic votes (we reproduced the results of the Polish national news broadcaster). Inauthentic content can take other forms, e.g., deep fakes were recently used for political disinformation in India and the U.S. and our study shows that thousands of Twitter polls gauging support for the 2016 and 2020 U.S. presidential candidates were heavily biased and likely manipulated in favor of one candidate, Donald Trump. We show that these biases result in amplification of the voices of privileged groups, i.e., old males. Such partisan and demographic biases among social polls have potentially serious consequences, including voter fraud beliefs. Unfortunately, the public is not typically informed about identified inauthenticity and is instead broadly exposed to inauthentic signals disguised as authentic ones. We argue that the information about provenance of political content that can be used for spreading disinformation is crucial to democratic processes. To avoid disinformation and misinformation, we call for more transparency about biases and inauthenticity of politically-relevant social media content.
From Fragmented Legislation to Global Alignment
The global landscape of AI technologies requires thinking through not just how to mitigate risk at city, state, and local levels but also federally and internationally. A federal office where these important issues can be considered together would be more effective than current ad-hoc responses. The needs for standards, transparency, and incentives for accountable AI discussed here motivate the establishment of a federal office to manage these complexities and the recent Brooking Institute report argues for cooperation and alignment of the U.S. and the European Union’s AI legislation. We propose the establishment of a new federal office to oversee AI systems by examining how complex technologies have been successfully regulated at the federal level. Specifically, we draw parallels to the following accountability structures and industries: the existing regulation for the medical device industry and the pharmaceutical industry (regulated by the FDA), the proposed legislation for AI in the European Union (the AI Act), and the existing U.S. anti-discrimination legislation. For instance, we advocate for classification of deployment types by risk levels, which resembles the FDA regulation and the AI Act proposals (Figure 1).
You will find details of our recommendations in the white paper. Please cite it as:
Przemyslaw Grabowicz, Nicholas Perello, and Yair Zick. “Towards an AI Accountability Policy”. https://www.regulations.gov/comment/NTIA-2023-0005-1424, 2023