AutoMTL: A Programming Framework for Automating Efficient Multi-Task Learning
By
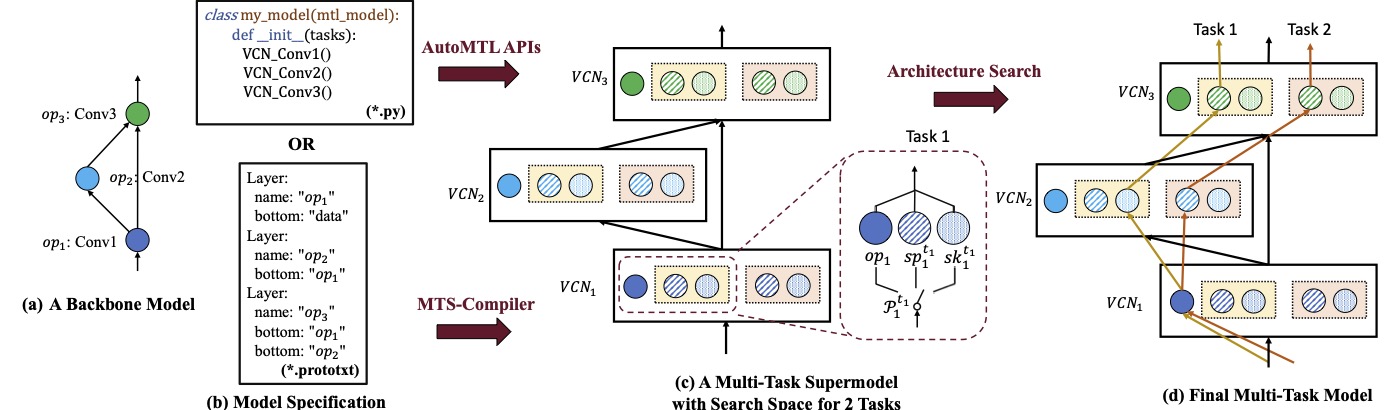
Full Abstract
Multi-task learning (MTL) jointly learns a set of tasks by sharing parameters among tasks. It is a promising approach for reducing storage costs while improving task accuracy for many computer vision tasks. The effective adoption of MTL faces two main challenges. The first challenge is to determine what parameters to share across tasks to optimize for both memory efficiency and task accuracy. The second challenge is to automatically apply MTL algorithms to an arbitrary CNN backbone without requiring time-consuming manual re-implementation and significant domain expertise. This paper addresses the challenges by developing the first programming framework AutoMTL that automates efficient MTL model development for vision tasks. AutoMTL takes as inputs an arbitrary backbone convolutional neural network (CNN) and a set of tasks to learn, and automatically produces a multi-task model that achieves high accuracy and small memory footprint simultaneously. Experiments on three popular MTL benchmarks (CityScapes, NYUv2, Tiny-Taskonomy) demonstrate the effectiveness of AutoMTL over state-of-the-art approaches as well as the generalizability of AutoMTL across CNNs. AutoMTL is open-sourced and available at https://github.com/zhanglijun95/AutoMTL.
General Summary
The effective adoption of MTL faces two main challenges. The first challenge is the resource-efficient architecture design–that is, to determine what parameters of a backbone model to share across tasks to optimize for both resource efficiency and task accuracy. Many prior works rely on manually-designed MTL model architectures which share several initial layers and then branch out at an ad hoc point for all tasks. They often result in unsatisfactory solutions due to the enormous architecture search space. Several recent efforts shift towards learning to share parameters across tasks. They embed policy-learning components into a backbone CNN and train the policy to determine which blocks in the network should be shared across which task or where to branch out for different tasks. Their architecture search spaces lack the flexibility to dynamically adjust model capacity based on given tasks, leading to sub-optimal solutions as the number of tasks grows.
The second major challenge is the automation. Manual architecture design calls for significant domain expertise when tweaking neural network architectures for every possible combination of learning tasks. Although neural architecture search (NAS)-based approaches automate the model design to some extent, the implementation of these works is deeply coupled with a specific backbone model. Some of them could theoretically support broader types of CNNs. They, however, require significant manual efforts and expertise to re-implement the proposed algorithms whenever the backbone changes. Our user study suggests that it takes machine learning practitioners with proficient PyTorch skills 20 to 40 hours to re-implement Adashare, a state-of-the-art NAS-based MTL approach, on a MobileNet backbone. The learning curve is expected to be much longer and more difficult for general programmers with less ML expertise. The lack of automation prohibits the effective adoption of MTL in practice.
In this paper, we address the two challenges by developing AutoMTL, the first programming framework that automates resource-efficient MTL model development for vision tasks. AutoMTL takes as inputs an arbitrary backbone CNN and a set of tasks to learn, and then produces a multi-task model that achieves high task accuracy and small memory footprint (measured by the number of parameters). AutoMTL features a source-to-source compiler that automatically transforms a user-provided backbone CNN to a supermodel that encodes the multi-task architecture search space in the operator-level granularity. It also offers a set of PyTorch-based APIs to allow users to flexibly specify the input backbone model. AutoMTL then performs gradient-based architecture searches on the supermodel to identify the optimal sharing patterns among tasks. Our experiments on several MTL benchmarks with a different number of tasks show that AutoMTL could produce efficient multi-task models with smaller memory footprint and higher task accuracy compared to state-of-the-art methods. AutoMTL also automatically supports arbitrary CNN backbones without any re-implementation efforts and improves the accessibility of MTL to general programmers.
Technical Summary
Given a backbone model, a user can specify the model using either the AutoMTL APIs or in the prototxt format. The model specification will be parsed by the MTS-compiler to generate a multi-task supermodel that encodes the entire search space. AutoMTL then identifies the optimal multi-task model architecture from the supermodel using gradient-based architecture search algorithms implemented in the Architecture Search component. AutoMTL supports the model specification in the format of prototxt as it is general enough to support various CNN architectures and also simple for our compiler to analyze. prototxt files are serialized using Google’s Protocol Buffers serialization library. AutoMTL API is currently implemented on top of PyTorch.
Link to project website
https://github.com/zhanglijun95/AutoMTL
Link to Paper