Regulating Face Recognition Technology: An Introduction
By
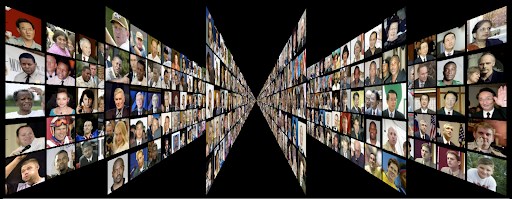
Face recognition technology, the ability for computers to identify people from photos or videos of their faces, has become increasingly controversial in the last few years. The software is rapidly becoming more common in applications from police work and surveillance to smart phone access, entertainment applications, and even medical diagnosis. There are those who wish to ban the technology completely based on its current misuse, and its great potential for future misuse. There are others who consider it essential to critical law enforcement applications for the good of society. Still others acknowledge there are problems, but believe those problems can be fixed by improving the technology. In this post, I will try to frame certain key issues that arise in discussions of the technology and how to regulate it. This post just scratches the surface. Many issues such as the privacy implications of face recognition, are not addressed here.
To delve into these issues, I’ll start with some of the pros and cons of the technology. Then I’ll talk about some common misconceptions that may affect how people think about the management of face recognition. My goal is to provide a few ideas that may help you come to your own conclusions about how society might manage this complex multi-faceted technology.
Pros and Cons
Face recognition (and related areas of face image analysis) and its role in society have been changing rapidly over the last 10 years. The popular press is filled with stories, not only about advances in the technology, but also about serious concerns with its use. As background for this post, consider the following developments about face recognition technology that have been widely publicized in the last few years. I split them into the categories negative, neutral, and positive, reflecting my own personal judgment of their impact on society (others may disagree):
Negative
- In January, 2020, Robert Williams of Detroit, Michigan, was misidentified by a face recognition algorithm and subsequently arrested for a crime he did not commit. Since then, other false arrests have been recorded. This is particularly concerning for Black citizens, both because of the technology’s poorer performance on those with darker skin, and also because of the history of unequal treatment of Blacks in law enforcement.
https://www.aclu.org/news/privacy-technology/i-did-nothing-wrong-i-was-arrested-anyway/ - The Chinese government has developed an extensive tracking system that uses face recognition technology, in conjunction with other tracking technology, to perform widespread surveillance on its citizens. Many civil rights groups worry that elements of such a system are likely to emerge in the United States if we do not take aggressive action to prevent it.
https://fortune.com/2020/11/03/china-surveillance-system-backlash-worlds-largest/
Neutral
- Face recognition is now widely used in personal cell phone applications, such as unlocking your phone. While this could be considered a positive development, I have rated it “neutral” since there are many existing alternatives to this technology, such as fingerprint recognition, and typed digital passwords. Thus, the added value to society of such a technology is limited.
- Face filtering applications that modify the appearance of faces in photographs are now widespread. While one can argue about the pros and cons of such technology, it is generally used for entertainment and recreational purposes. It is usually neither particularly useful nor harmful to society.
Positive
- Face recognition has been used to identify victims of child abuse and child abusers, helping law enforcement solve difficult cases. Such applications are of course complex trade-offs between the benefits of apprehending criminals and the civil rights of innocent people. However, as the New York Times says, “it’s a powerful use case for the … technology.”
https://www.nytimes.com/2020/02/07/business/clearview-facial-recognition-child-sexual-abuse.html - A less well-known application of face recognition technology is the automatic screening of medical conditions, such as hyperthyroidism and acromegaly, both of which are medical conditions that can change the appearance of a person’s face. While still in the developmental stages, such technology could provide inexpensive screening for a number of medical conditions.
This is just a small sampling of the pros and cons of face recognition and its rapidly increasing adoption in the United States. Not surprisingly, there are many who advocate for a complete ban of face recognition technology, while others argue that the benefits far outweigh the risks.
One thing that I’ve encountered in my discussions about face recognition is the idea that if the technology is sufficiently accurate, it will be unlikely to have negative effects. The thinking is that if the technology makes no mistakes, then it will not create problems. However, this idea is flawed in at least two ways. First, for reasons I will discuss below, the technology will never be error free. Second, even without making misidentification errors, there are side effects of using the technology, such as violations of privacy, that are distinct from issues of accuracy. Thus, if we are going to live with this technology, we need to learn to manage it, warts and all. But before getting to that, let’s start with a closer look at the accuracy of the technology.
There is no question that there have been tremendous advances in face recognition accuracy in the last two decades. The UMass Amherst face recognition benchmark, called Labeled Faces in the Wild, measures the accuracy of face recognition algorithms on a very simple task—deciding whether two face images are the same person (see Figure below). When we released the benchmark in 2007, no algorithm achieved better than 75 percent accuracy on the benchmark’s test suite. Today, dozens of different algorithms achieve well over 99 percent.
Will face recognition systems ever be error free?
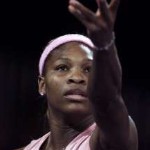
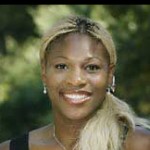
Same or different? A common face recognition task, known as verification, asks whether two photographs represent the same person. This tests a computer’s ability to recognize a person under changes in many factors, including lighting, pose, hairstyle, clothing, makeup, occlusions, and facial expression. (Both pictures show tennis pro Serena WIlliams.)
Does this mean that face recognition algorithms are, or will soon be, error free? The answer to this is a resounding “No!”. The critical thing to understand about face recognition is that the circumstances of the problem can render it arbitrarily difficult. That is, no matter how sophisticated the technology, there will always be cases that are difficult to get right. Here are two examples of how face recognition can vary from relatively easy to extremely difficult:
- Size of the gallery. In most face recognition scenarios, an image is compared against a group of individuals for a possible match. This group is known as the gallery, and it represents a set of people who have already been photographed. In some cases, the gallery may be relatively small. For example, a company may make a gallery of the 20 employees who work in a particular building, so that someone at the entrance can be compared against those people for permission to enter. Given that there are only 20 possible matches, the likelihood that a random person trying to enter the building will look very similar to one of the employees is fairly low. Hence, such a system will typically exhibit few false matches.
However, consider a federal database with millions of different people in the gallery. In this case, when a new photo is entered for a database match, it is likely that there will be a number of people in the database who look very similar to the person in the new photo. Thus, the chance of producing a false match becomes much higher. This problem gets worse as the galleries become larger and larger.
- Image quality. Even more difficult to overcome than the size of the gallery is the issue of image quality. As any person knows, the human ability to recognize people and objects continues to drop as the amount of light in a scene drops. Computer recognition is no different. As the lighting of a scene becomes lower and lower, recognition accuracy rates will continue to fall. In the limit, as the image becomes almost completely black, it can become arbitrarily difficult to recognize a person, either by computer or by human. This is not a complicated idea, and yet is frequently overlooked in the discussion of the technology. For example, if someone robs a store and tries to conceal their identity by turning off the lights, nothing prevents investigators from taking a poorly lit surveillance camera image and trying to get matches to a face database. For very poor images, we can never expect a face recognition algorithm to return accurate results. Laypeople who are using such systems and do not understand how the technology works may incorrectly assume that there is some “magic” the computer can do to overcome this poor image quality. Hence, they may see nothing wrong with putting a low-quality image into a face recognition system. This problem can be exacerbated by unrealistic TV shows and movies that show computers doing unrealistic “enhancement” of low quality images, an ability which does not exist and never will.
There are many other phenomena that can make face recognition difficult, such as twins, facial injuries, occlusions, make-up, disguise, backlighting, and so on. Next, we look at whether a computer can be taught to estimate its own accuracy.
Knowing what you don’t know
If a human is asked to name the person in a poorly lit image, they are likely to say something like, “I have no idea; the image is of such poor quality that I can’t tell who is in it.” The bad news about this is that they can’t tell you who’s in the image. But the good news is that people are, at least in this situation, aware that they can’t make a good guess. Unfortunately, many face recognition systems are not good at assessing their own confidence, or even worse, may not assess their own confidence at all.
For example, if I put a completely blank picture into a face recognition system and force it to tell me whether it is more similar to Alice or Bob, there may be no way for the system to communicate that it has no idea. It has essentially been forced to choose one or the other. Of course, either answer (Alice or Bob) is absurd.
Many commercial face recognition systems attempt to address this problem by producing a “confidence score” for any given result. For example, a system may output a confidence score of “95%”. But what exactly is this supposed to mean?
A common way to interpret such a confidence score is to say that, if I gather up all of the cases in which a system gives a 95% confidence score, then at least 95% of them should be correct. Such a system is said to be well calibrated. If commercial systems were, in fact, well calibrated, then we could rely on the confidence scores to make decisions about whether to act on the results of a system or not.
Unfortunately, good calibration appears to be an unsolved problem. It is not uncommon to see a face recognition system report 100% confidence and yet still make an error! Calibration is extremely difficult, since it depends on so many different factors that may vary from scene to scene. For example, imagine the poor lighting in a late night bar room scene. An algorithm may think it has a good match, but how confident is it? To calibrate such a system well for this situation it may need to be trained on this exact environment. And of course there are too many environments to do this effectively.
Of course, some day, we may be able to calibrate face recognition systems well, but at least to my knowledge, as someone who is up to date with the scientific literature, I am not aware of any well-calibrated face recognition systems. This is a serious drawback, since it makes it very difficult for users to assess the reliability of such systems.
How to manage face recognition technology
Given the inherent limitations described above, how should we manage face recognition technology? My colleagues and I recently wrote a white paper about this topic, in which we propose a federal organization that works like the Food and Drug Administration to regulate face recognition. While going over the details of that long document is beyond the scope of this post, here are some of the key takeaways.
- For any given face recognition application, define the risks and harms that can occur when mistakes are made. For example, in police work, errors in face recognition can result in false arrest, or worse, while in an entertainment application, like SnapChat, the consequences of errors are far less severe.
- Establish federal standards for testing that are commensurate with the risks as defined above. That is, for applications with minimal risks, there may be no need for exhaustive and expensive testing. However, for applications with serious negative impacts, a much higher testing and validation bar should be required.
- For high risk applications, measure real world performance over time, and make adjustments as needed. For example, when the FDA releases a new drug, they continue to collect data about negative side effects, and occasionally make a recall if they find worse-than-expected outcomes. Such real world performance tracking is essential since laboratory tests can never completely predict real-world performance.
These are just a few of the recommendations we make in our white paper, but it gives you the flavor of how one can manage a technology that may still have some flaws. While it is a laudable goal to eliminate all of the flaws, it is also necessary to understand that there will probably always be some problems with technology, and to adapt our management of it to handle these inevitable problems.