Equi-explanation Maps: Concise and Informative Global Summary Explanations
By
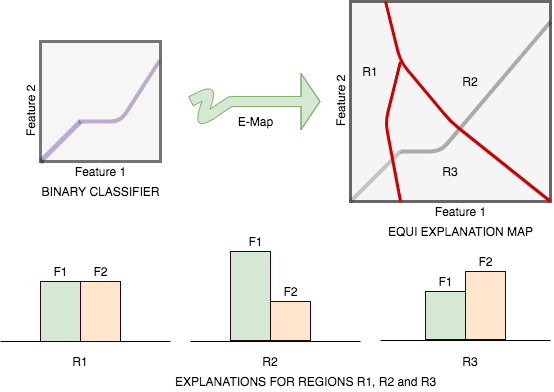
Full Abstract
We attempt to summarize the model logic of a black-box classification model in order to generate concise and informative global explanations. We propose equi-explanation maps, a new explanation data-structure that presents the region of interest as a union of equi-explanation subspaces along with their explanation vectors.
We then propose E-Map, a method to generate equi-explanation maps. We demonstrate the broad utility of our approach by generating equi-explanation maps for various binary classification models (Logistic Regression, SVM, MLP, and XGBoost) on the UCI Heart disease dataset and the Pima Indians diabetes dataset. Each subspace in our generated map is the union of d-dimensional hyper-cuboids which can be compactly represented for the sake of interpretability. For each of these subspaces, we present linear explanations assigning a weight to each explanation feature.
We justify the use of equi-explanation maps in comparison to other global explanation methods by evaluating in terms of interpretability, fidelity, and informativeness. A user study further corroborates the use of equi-explanation maps to generate compact and informative global explanations.
General Summary
We have seen an exponential rise of Explainable AI papers in recent years. Many of them address the task of global explanations, which is a summary of the model’s behavior across the feature space via explanations. There are two popular data structures used to present global explanations : representative instances and falling if-then lists. We propose equi-explanation maps, a new explanation data-structure that presents the region of interest as a union of equi-explanation subspaces along with their explanation vectors. We demonstrate the broad utility of our approach by generating equi-explanation maps for various binary classification models (Logistic Regression, SVM, MLP, and XGBoost) on the UCI Heart disease dataset and the Pima Indians diabetes dataset. A user study further corroborates the use of equi-explanation maps to generate compact and informative global explanations.
Link to Paper
https://dl.acm.org/doi/10.1145/3531146.3533112
Conference name
ACM Conference on Fairness, Accountability, and Transparency (ACM FAccT)]
Video
Short Video