Serendyze
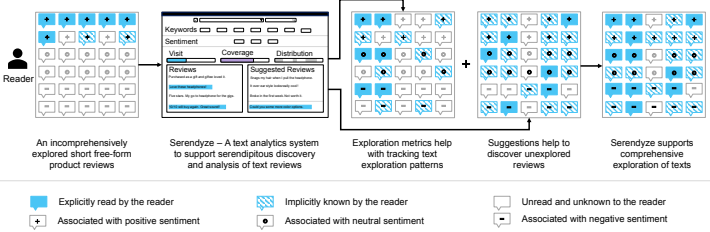
Led by: Mahmood Jasim, Christopher Collins, Ali Sarvghad, Narges Mahyar
Related Papers: Supporting Serendipitous Discovery and Balanced Analysis of Unstructured Text with Interaction-Driven Metrics and Bias-Mitigating Suggestions
CHI Talk
In this study, we investigate how supporting serendipitous discovery and analysis of short free-form texts, such as product review can encourage readers to explore texts more comprehensively prior to decision-making. We propose two interventions — Exploration Metrics that help readers understand and track their exploration patterns through visual indicators and a Bias Mitigation Model that maximizes knowledge discovery by suggesting readers sentiment and semantically diverse reviews. We designed, developed, and evaluated a text analytics system called Serendyze, where we integrated these interventions. We asked 100 crowd workers to use Serendyze to make purchase decisions based on product reviews. Our evaluation suggests that exploration metrics enable readers to efficiently cover more reviews in a balanced way, and suggestions from the bias mitigation model influence readers to make confident data-driven decisions. We discuss the role of user agency and trust in text-level analysis systems and their applicability in domains beyond review exploration
If you are interested in working on or learning more about this project, please contact Mahmood Jasim at mjasim@cs.umass.edu