Paper: Structural Credit Assignment in Neural Networks using Reinforcement Learning
By
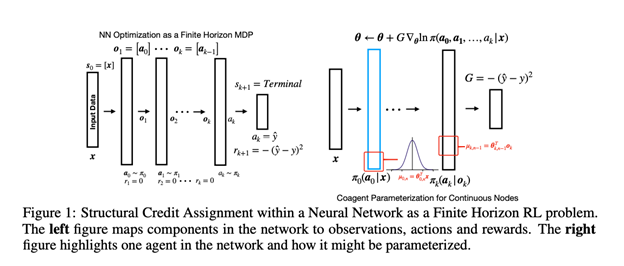
Consider an n x n Gaussian kernel matrix corresponding to n input points in d dimensions. We show that one In this work, we revisit REINFORCE and investigate if we can leverage other reinforcement learning approaches to improve learning. We formalize training a neural network as a finite-horizon reinforcement learning problem and discuss how this facilitates using ideas from reinforcement learning like off-policy learning. We show that the standard on-policy REINFORCE algorithm, even with variance reduction approaches, learns sub-optimal solutions. We introduce an off-policy approach, to facilitate reasoning about the greedy action for other agents and help overcome stochasticity in other agents. We conclude by showing that these networks of agents can be more robust to correlated samples when learning online.