Publications
Journal Publications Book Chapters In Proceedings Abstracts and Short Papers
Books
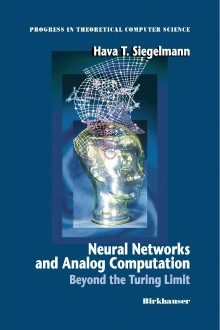
Neural Networks and Analog Computation: Beyond the Turing Limit
H.T. Siegelmann
Neural Networks and Analog Computation:
Beyond the Turing Limit, Birkhauser
Boston, December 1998
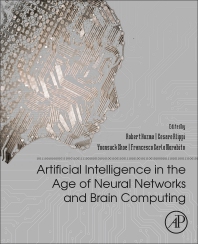
Artificial Intelligence in the Age of Neural Networks and Brain Computing
Robert Kozma, Cesare Alippi, Yoonsuck Choe, Francesco Morabito
Artificial Intelligence in the Age of Neural Networks and Brain Computing
November 2018
Journal Publications
- Yu, C., Rietman, E. A., Siegelmann, H. T., Cavaglia, M., & Tuszynski, J. A. (2021). Application of Thermodynamics and Protein–Protein Interaction Network Topology for Discovery of Potential New Treatments for Temporal Lobe Epilepsy. Applied Sciences, 11(17), 8059. https://doi.org/10.3390/app11178059
- Amgalan, A., Taylor, P., Mujica-Parodi, L.R. et al. Unique scales preserve self-similar integrate-and-fire functionality of neuronal clusters. Sci Rep 11, 5331 (2021). https://doi.org/10.1038/s41598-021-82461-4
- B. Tsuda, K. M. Tye, H. T. Siegelmann, T. J. Sejnowski, “A modeling framework for adaptive lifelong learning with transfer and savings through gating in the prefrontal cortex,” Proceedings of the National Academy of Sciences, November 2020.
- G.M. van de Ven, H. T. Siegelmann, A. S. Tolias Brain-inspired replay for continual learning with artificial neural networks. Nature Communications, 11, Article number: 4069, August 2020.
- M. Shifrin and H.T. Siegelmann, “Near Optimal Insulin Treatment for Diabetes Patients: A machine learning approach,” Artificial Intelligence in Medicine (AIIM), 107, July 2020.
- E. A. Rietman, S. Taylor, H.T. Siegelmann, M.A. Deriu, M. Cavaglia, and J.A. Tuszynski, (2020) “Using the Gibbs Function as a Measure of Human Brain Development Trends from Fetal Stage to Advanced Age,” International Journal of Molecular Sciences 21(3), Feature Papers in Molecular Biophysics,” February 2020.
- Brant, Elizabeth J., et al. “Personalized therapy design for systemic lupus erythematosus based on the analysis of protein-protein interaction networks.” Plos one 15.3 (2020): e0226883.
- D. Patel, H. Hazan, D.J. Saunders, H. Siegelmann, R. Kozma (2019). Improved robustness of reinforcement learning policies upon conversion to spiking neuronal network platforms applied to ATARI games. Neural Networks, 120, 108-115. https://arxiv.org/abs/1903.11012
- D.J. Saunders, D. Patel, H. Hazan, H.T. Siegelmann, T. Kozma (2019). Locally Connected Spiking Neural Networks for Unsupervised Feature Learning. Neural Networks, 119, pp. 332-340. https://arxiv.org/abs/1904.06269
- de Bruyn Kops, S. M., et al. “Unsupervised Machine Learning to Teach Fluid Dynamicists to Think in 15 Dimensions.” arXiv (2019): arXiv-1907.
- Hazan, H., Saunders, D. J., Sanghavi, D. T., Siegelmann, H., & Kozma, R. (2019). Lattice Map Spiking Neural Networks (LM-SNNs) for Clustering and Classifying Image Data, Annals of Mathematics and Artificial Intelligence, pp. 1-24. https://arxiv.org/pdf/1906.11826.pdf
- Kenney, Jack, et al. “Deep Learning Regression of VLSI Plasma Etch Metrology.” arXiv preprint arXiv:1910.10067 (2019).
- Heck, Detlef H., Robert Kozma, and Leslie M. Kay. “The rhythm of memory: how breathing shapes memory function.” Journal of Neurophysiology 122.2 (2019): 563-571.
- Davis, Jeffery Jonathan Joshua, Robert Kozma, and Florian Schübeler. “Stress Reduction, Relaxation, and Meditative States Using Psychophysiological Measurements Based on Biofeedback Systems via HRV and EEG.” (2019).
- Davis, Jeffery Jonathan Joshua, and Robert Kozma. “Movie-Making of Spatiotemporal Dynamics in Complex Systems.” (2019).
- Janson, Svante, et al. “A modified bootstrap percolation on a random graph coupled with a lattice.” Discrete Applied Mathematics 258 (2019): 152-165.
- Golas, Stefan M., et al. “Gibbs free energy of protein-protein interactions correlates with ATP production in cancer cells.” Journal of Biological Physics 45.4 (2019): 423-430.
- H. Hazan, D.J. Saunders, H. Khan, D. Patel, S.T. Sanghavi, H.T. Siegelmann, R. Kozma, BindsNET: A Machine Learning-Oriented Spiking Neural Networks Library in Python, Frontiers in Neuroinformatics, Dec. 2018. (doi: 10.3389/fninf.2018.00089)
- Hossain, Gahangir, Mark H. Myers, and Robert Kozma. “Spatial directionality found in frontal-parietal attentional networks.” Neuroscience journal 2018 (2018).
- Myers, Mark H., and Robert Kozma. “Mesoscopic neuron population modeling of normal/epileptic brain dynamics.” Cognitive neurodynamics 12.2 (2018): 211-223.
- Kozma, Robert, and Joshua JJ Davis. “Why do phase transitions matter in minds?.” Journal of Consciousness Studies 25.1-2 (2018): 131-150.
- Bressler, Steven, Leslie Kay, and G. Vitiello. “Freeman neurodynamics: The past 25 years.” Journal of Consciousness Studies 25.1-2 (2018): 13-32.
- S. H. McGuire, E. A. Rietman, H. Siegelmann & J. A. Tuszynski, “Gibbs free energy as a measure of complexity correlates with time within C. elegans embryonic development,” Journal of Biological Physics, Dec;43(4):551-563, EPub: September 19th 2017. https://doi.org/10.1007/s10867-017-9469-0
- Burroni, P. Taylor, C. Corey, T. Vechnadze, H.T. Siegelmann, “Energetic Constraints Produce Self-sustained Oscillatory Dynamics in Neuronal Networks,” Frontiers in Neuroscience, 11(80), February 2017, 14 pages. https://doi.org/10.3389/fnins.2017.00080
- Kozma, Robert, and Walter J. Freeman. “Cinematic operation of the cerebral cortex interpreted via critical transitions in self-organized dynamic systems.” Frontiers in systems neuroscience 11 (2017): 10.
- Heck, Detlef H., et al. “Breathing as a fundamental rhythm of brain function.” Frontiers in neural circuits 10 (2017): 115.
- Kozma, Robert, and Raymond Noack. “Freeman’s intentional neurodynamics.” Intentional neurodynamics in transition: The dynamical legacy of Walter Jackson Freeman, special issue of Chaos and Complexity Letters 11.1 (2017): 93-103.
- Rietman, Edward A., et al. “Personalized anticancer therapy selection using molecular landscape topology and thermodynamics.” Oncotarget 8.12 (2017): 18735.
- Lee, Minho, Steven Bressler, and Robert Kozma. “Advances in Cognitive Engineering Using Neural Networks.” Neural Networks: the Official Journal of the International Neural Network Society 92 (2017): 1-2.
- Kay, Leslie M., and Robert Kozma. “Walter J. Freeman: A Tribute.” Neuron 94.4 (2017): 705-707.
- Rietman, Edward A., and Jack A. Tuszynski. “Thermodynamics and Cancer Dormancy: A Perspective.” Tumor Dormancy and Recurrence. Humana Press, Cham, 2017. 61-79.
- Capolupo, Antonio, et al. “Bessel-like functional distributions in brain average evoked potentials.” Journal of integrative neuroscience 16.s1 (2017): S85-S98.
- Rietman, Edward A., et al. “Thermodynamic measures of cancer: Gibbs free energy and entropy of protein–protein interactions.” Journal of biological physics 42.3 (2016): 339-350.
- Janson, Svante, et al. “Bootstrap percolation on a random graph coupled with a lattice.” Electronic Journal of Combinatorics (2016).
- P. Taylor, J.N. Hobbs, J Burroni, H.T. Siegelmann, “The global landscape of cognition: hierarchical aggregation as an organizational principle of human cortical networks and functions,” Nature Scientific Reports Dec 2015.
- P. Taylor, Z. He, N. Bilgrien, H.T. Siegelmann, “Human strategies for multitasking, search, and control improved via real-time memory aid for gaze location,” Frontiers in ICT 2:15, Sept 2015. doi: 10.3389/fict.2015.00015
- P. Taylor, Z. He, N. Bilgrien, H.T. Siegelmann, “EyeFrame: real-time memory aid improves human multitasking via domain-general eye tracking procedures,” Frontiers in ICT 2:17, Sept 2015. doi: 10.3389/fict.2015.00017
- J. Cabessa and H. T. Siegelmann, “The Super-Turing Computational Power of Plastic Recurrent Neural Networks,” International Journal of Neural Systems 24(8) 2014.
- Hava Siegelmann and Rudolf Freund, “Report on the 13th International Conference on Unconventional Computation and Natural Computation (UCNC’14) Ontario, Canada, July 14-18, 2014,” Bulletin of European Association for Theoretical Computer Science (EATCS), number 114, October 2014, pp. 265-269. http://www.eatcs.org/images/bulletin/beatcs114.pdf
- A. Tal, N. Peled and H. T. Siegelmann, “Biologically inspired load balancing mechanism in neocortical competitive learning,” Frontiers in Neural Circuits. March 2014 | doi: 10.3389/fncir.2014.00018.
- D. Nowicki, P. Verga and H.T. Siegelmann, “Modeling Reconsolidation in Kernel Associative Memory,” PLoS ONE. Aug 2013, 8(8): e68189. doi:10.1371/journal.pone.0068189
- H.T. Siegelmann, “Turing on Super-Turing and Adaptivity”. J. Progress in Biophysics & Molecular Biology. April (Sep) 2013, 113(1):117-26. doi: 10.1016/j.pbiomolbio.2013.03.013.
- E. Kagan, A. Rybalov, H. T. Siegelmann, and R. Yager, “Probability-generated aggregators,” International Journal of Intelligent System. July 2013, 28(7): 709-727.
- J. Cabessa and H. T. Siegelmann, “The Computational Power of Interactive Recurrent Neural Networks,” Neural Computation. April 2012, 24(4): 996-1019.
- Frederick C. Harris, Jr., Jeffrey L. Krichmar, Hava Siegelmann, Hiroaki Wagatsuma, “Biologically-Inspired Human-Robot Interactions – Developing More Natural Ways to Communicate with our Machines,” IEEE Transactions on Autonomous Mental Development 2012 Special issue 4(3): 190-191.
- Jean-Philippe Thivierge, Ali Minai, Hava Siegelmann, Cesare Alippi, Michael Geourgiopoulos, “A year of neural network research: Special Issue on the 2011 International Joint Conference on Neural Networks,” Neural Networks Special issue, Volume 32, Pages 1-2, 2012.
- H.T. Siegelmann, “Addiction as a Dynamical Rationality Disorder,” Frontiers of Electrical and Electronic Engineering (FEE) in China. 1(6), 2011:151-158.
- L. Glass and H.T. Siegelmann, “Logical and symbolic analysis of robust biological dynamics,” Current Opinion in Genetics & Development 20, 2010: 644-649.
- M.M. Olsen, K. Harrington, H. T. Siegelmann, “Conspecific Emotional Cooperation Biases Population Dynamics: A Cellular Automata Approach,” International Journal of Natural Computing Research 1(3) 2010: 51-65.
- H. T. Siegelmann and L.E. Holtzman, “Neuronal integration of dynamic sources: Bayesian learning and Bayesian inference,” Chaos: Focus issue: Intrinsic and Designed Computation: Information Processing in Dynamical Systems 20 (3): DOI: 10.1063/1.3491237, September 2010. (7 pages)
- D. Nowicki and H.T. Siegelmann, “Flexible Kernel Memory,” PLOS One 5: e10955, June 2010. http://www.plosone.org/article/info%3Adoi%2F10.1371%2Fjournal.pone.0010955 (18 pages)
- M.M. Olsen, N. Siegelmann-Danieli, H.T. Siegelmann. “Dynamic Computational Model Suggests that Cellular Citizenship is Fundamental for Selective Tumor Apoptosis,” PLoS One 5(5):e10637, May 2010. http://www.plosone.org/article/info:doi%2F10.1371%2Fjournal.pone.0010637 (6 pages)
- Siegelmann, H.T., “Complex Systems Science and Brain Dynamics: A Frontiers in Computational Neuroscience Special Topic,” 2010, doi: 10.3389/fncom. 2010. 00007
- K. Tu, D. G. Cooper, H. T. Siegelmann, “Memory Reconsolidation for Natural Language Processing,” Cognitive Neurodynamics 3(4), 2009: 365-372.
- A. Z. Pietrzykowski, R. M. Friesen, G. E. Martin, S.I. Puig, C. L. Nowak, P. M. Wynne, H. T. Siegelmann, S. N. Treistman, “Post-transcriptional regulation of BK channel splice variant stability by miR-9 underlies neuroadaptation to alcohol,” Neuron 59, July 2008: 274-287.
- Lu, S., Becker, K.A., Hagen, M.J., Yan, H., Roberts, A.L., Mathews, L.A., Schneider, S.S., Siegelmann, H.T., Tirrell, S.M., MacBeth, K.J., Blanchard, J.L. and Jerry, D.J., “Transcriptional responses to estrogen and progesterone in Mammary gland identify networks regulating p53 activity,” Endocrinology 149(10), June 2008: 4809-4820.
- H.T. Siegelmann, “Analog-Symbolic Memory that Tracks via Reconsolidation,” Physica D: Nonlinear Phenomena 237 (9), 2008: 1207-1214.
- M.M. Olsen, N. Siegelmann-Danieli and H.T. Siegelmann, “Robust Artificial Life Via Artificial Programmed Death,” Artificial Intelligence 172(6-7), April 2008: 884-898.
- F. Roth, H. Siegelmann, R. J. Douglas. “The Self-Construction and -Repair of a Foraging Organism by Explicitly Specified Development from a Single Cell,” Artificial Life 13(4), 2007: 347-368.
- S. Sivan, O. Filo and H. Siegelman, “Application of Expert Networks for Predicting Proteins Secondary Structure,” Biomolecular Engineering 24(2), June 2007: 237-243.
- W. Bush and H.T. Siegelmann, “Circadian Synchronicity in Networks of Protein Rhythm Driven Neurons,” Complexity 12(1), September/October 2006: 67-72.
- T. Leise and H.T. Siegelmann, “Dynamics of a multistage circadian system,” Journal of Biological Rhythms 21(4), August 2006: 314-323.
- L. Glass, T. J. Perkins, J. Mason, H. T. Siegelmann and R. Edwards, “Chaotic Dynamics in an Electronic Model of a Genetic Network,” Journal of Statistical Physics 121(5-6), November 2005: 969-994.
- O. Loureiro, and H. Siegelmann, “Introducing an Active Cluster-Based Information Retrieval Paradigm,” Journal of the American Society for Information Science and Technology 56(10), August 2005: 1024-1030.
- Roitershtein, A. Ben-Hur and H.T. Siegelmann “On probabilistic analog automata,” Theoretical Computer Science 320(2-3), June 2004: 449-464.
- A. Ben-Hur and H.T. Siegelmann, “Computing with Gene Networks,” Chaos 14(1), March 2004: 145-151.
- A. Ben-Hur, J. Feinberg, S. Fishman and H. T. Siegelmann, “Random matrix theory for the analysis of the performance of an analog computer: a scaling theory,” Physics Letters A. 323(3-4), March 2004: 204-209.
- A. Ben-Hur, J. Feinberg, S. Fishman and H. T. Siegelmann, “Probabilistic analysis of a differential equation for linear programming,” Journal of Complexity 19(4), August 2003: 474-510.
- J. P. Neto, H. T. Siegelmann, and J. F. Costa, “Symbolic processing in neural networks,” Journal of the Brazilian Computer Society 8(3), July 2003: 58-70.
- H. T. Siegelmann, “Neural and Super-Turing Computing,” Minds and Machines
13(1), February 2003: 103-114. - S Eldar, H. T. Siegelmann, D. Buzaglo, I. Matter, A. Cohen, E. Sabo, J. Abrahamson, “Conversion of Laparoscopic Cholecystectomy to open cholecystectomy in acute cholecystitis: Artificial neural networks improve the prediction of conversion,” World Journal of Surgery 26(1), Jan 2002: 79-85.
- A. Ben-Hur, H.T. Siegelmann and S. Fishman, “A theory of complexity for continuous time dynamics,” Journal of Complexity 18(1), 2002: 51-86.
- A. Ben-Hur, D. Horn, H.T. Siegelmann and V. Vapnik, “Support vector clustering,” Journal of Machine Learning Research 2, 2001: 125-137.
- H. T. Siegelmann, “Neural Computing,” Bulletin of the European Association of Theoretical Computer Science (EATCS) 73, 2001: 107-130.
- H. T. Siegelmann A., Ben-Hur, S. Fishman, “Comments on Attractor Computing,” International Journal of Computing Anticipatory Systems 6, 1999. (from CASY’99 International Conference on Computing Anticipatory Systems, Belgium, August 9-14, D.M. Dubois editor)
- R. Edwards, H.T. Siegelmann, K. Aziza and L. Glass, “Symbolic dynamics and computation in model gene networks”, Chaos 11(1), 2001: 160-169.
- H. Lipson and H.T. Siegelmann, “Geometric Neurons for Clustering,” Neural Computation 12(10), August 2000: 2331-2353.
- D. Lange, H.T. Siegelmann, H. Pratt, and G.F. Inbar, “Overcoming Selective Ensemble Averaging: Unsupervised Identification of Event Related Brain Potentials.” IEEE Transactions on Biomedical Engineering 47(6), June 2000: 822-826.
- H. Karniely and H.T. Siegelmann, “Sensor Registration Using Neural Networks,” IEEE transactions on Aerospace and Electronic Systems 36(1), 2000: 85-98.
- H. T. Siegelmann, “Stochastic Analog Networks and Computational Complexity,” Journal of Complexity 15(4), 1999: 451-475.
- T. Siegelmann, A. Ben-Hur and S. Fishman, “Computational Complexity for Continuous Time Dynamics,” Physical Review Letters, 83(7), 1999: 1463-1466.
- H. T. Siegelmann and M. Margenstern, “Nine Neurons Suffice for Turing Universality,” Neural Networks 12, 1999: 593-600.
- Gavaldà and H.T. Siegelmann, “Discontinuities in Recurrent Neural Networks,” Neural Computation 11(3), April 1999: 715-745.
- H. T. Siegelmann and S. Fishman, “Computation by Dynamical Systems,” Physica D 120, 1998 (1-2): 214-235.
- Galperin, Y. Kimhi, E. Nissan, and H.T. Siegelmann, “FULECON’s Heuristics, their Rationale, and their Representations,” The New Review of Applied Expert Systems 4, 1998: 163-176.
- H. T. Siegelmann, E. Nissan, and A. Galperin, “A Novel Neural/Symbolic Hybrid Approach to Heuristically Optimized Fuel Allocation and Automated Revision of Heuristics in Nuclear Engineering,” Advances in Engineering Software 28(9), 1997: 581-592.
- J. L. Balcázar, R. Gavaldà, and H.T. Siegelmann, “Computational Power of Neural Networks: A Characterization in Terms of Kolmogorov Complexity,” IEEE Transactions on Information Theory 43(4), July 1997: 1175-1183.
- J. P. Neto, H.T. Siegelmann, and J.F. Costa, “Implementation of Programming Languages with Neural Nets,” International Journal of Computing Anticipatory Systems 1, 1997: 201-208
- H. T. Siegelmann, B.G. Horne, and C.L.Giles, “Computational Capabilities of Recurrent NARX Neural Networks,” IEEE Transaction on Systems, Man and Cybernetics – part B: Cybernetics 27(2), 1997: 208-215.
- E. Nissan, H.T. Siegelmann, A. Galperin, and S. Kimhi, “Upgrading Automation for Nuclear Fuel In-Core Management: From the Symbolic Generation of Configurations, to the Neural Adaptation of Heuristics,” Engineering with Computers 13(1), 1997: 1-19.
- O. Frieder and H.T. Siegelmann, “Document Allocation: A Genetic Algorithm Approach,” IEEE Transactions on Knowledge and Data Engineering 9(4), 1997: 640-642. (Work described in American Scientist)
- H. T. Siegelmann and C.L. Giles, “The Complexity of Language Recognition by Neural Networks,” Journal of Neurocomputing, special Issue on Recurrent Networks for Sequence Processing, Editors: M. Gori, M. Mozer, A.H. Tsoi, W. Watrous, 15(3-4), 1997: 327-345.
- H. T. Siegelmann, “On NIL: The Software Constructor of Neural Networks,” Parallel Processing Letters 6(4), 1996: 575-582.
- H. T. Siegelmann, “The Simple Dynamics of Super Turing Theories,” Theoretical Computer Science (special issue on UMC) 168(2), 1996: 461-472.
- H. T. Siegelmann, “Recurrent Neural Networks and Finite Automata,” Journal of Computational Intelligence 12(4), 1996: 567-574.
- J. Kilian and H.T. Siegelmann, “The Dynamic Universality of Sigmoidal Neural Networks,” Information and Computation 128(1), 1996: 45-56.
- H. T. Siegelmann, “Analog Computational Power, Technical comment,” Science 271(19), January 1996: 373.
- B. DasGupta, H.T. Siegelmann and E. Sontag, “On the Complexity of Training Neural Networks with Continuous Activation Functions,” IEEE Transactions on Neural Networks 6(6), 1995: 1490-1504.
- H. T. Siegelmann, “Computation Beyond the Turing Limit,” Science 238(28), April 1995: 632-637. (Work received wide media attention, and was mentioned as founding the field of HyperComputation.)
- H. T. Siegelmann and E.D. Sontag, “Computational Power of Neural Networks,” Journal of Computer System Sciences 50(1), 1995: 132-150. (Work described as most fundamental theorem about neural networks in Simon Haykin’s book of Neural Networks.)
- H. T. Siegelmann and E.D. Sontag, “Analog Computation via Neural Networks,” Theoretical Computer Science 131, 1994: 331-360. (Work described as the fundamental theorem differentiating neural networks from classical computers in Simon Haykin’s book of Neural Networks; is cited in the field and in the media.)
- H. T. Siegelmann and E.D. Sontag, “Turing Computability with Neural Networks,” Applied Mathematics Letters 4(6), 1991: 77-80.
Book Chapters
- H.T. Siegelmann and R. Kozma, “Associative Learning,” UNESCO Encyclopedia of Life Support Systems (EOLSS), Vol. Computational Intelligence, (Eds) H. Ishibuchi, UNESCO EOLSS Press, New York, 2015.
- E. Kagan, A. Rybalov, A. Sela, H. Siegelmann, J. Steshenko, “Probabilistic control and swarm dynamics in mobile robots and ants,” in Biologically-Inspired Techniques for Knowledge Discovery and Data Mining, S. A. Burki, G. Dobbie and Y. S. Koh (eds.), pp.11-47, 2014 http://www.igi-global.com/chapter/probabilistic-control-and-swarm-dynamics-in-mobile-robots-and-ants/110453
- H. T. Siegelmann, “Super Turing As a Cognitive Reality,” (Chapter 21) in Consciousness: Its Nature and Functions, Shulamith Kreitler and Oded Maimon (eds), Nova Publishers, 2012, Hauppauge, NY: 401-410.
- K. I. Harrington and H. T. Siegelmann, “Adaptive Multi-modal Sensors,” in 50 years of Artificial Intelligence, Lungarella, F. Iida, J. Bongard, R. Pfeifer (eds.) Springer 2007: 264-173.
- Bhaskar DasGupta, Derong Liu and Hava Siegelmann, “Neural Networks,” in Handbook on Approximation Algorithms and Metaheuristics, Teofilo F. Gonzalez (editor), Chapman & Hall/CRC (Computer & Information Science Series, series editor: Sartaj Sahni) 2007: 22-1—22-14.
- H. T. Siegelmann, “Neural Computing,” in Current Trends in Theoretical Computer Science: The Challenge of the New Century, G. Paun, G. Rozenberg, A. Salomaa (eds), 2004.
- H.T. Siegelmann, “Neural Automata and Computational Complexity,” in Handbook of Brain Theory and Neural Networks, M.A. Arbib (ed.), Birkhauser Boston, 2002.
- H.T. Siegelmann, “Universal Computation and Super-Turing Capabilities,” in Field Guide to Dynamical Recurrent Networks, J.F. Kolen and S.C. Kremer (eds.), IEEE Press, 2001:143-151.
- A. Ben-Hur and H.T. Siegelmann, “Computation in gene networks,” in Machines, Computations and Universality (MCU), Lecture Notes in Computer Science, M. Margenstern and Y. Rogozhin (Eds.) 2055, 2001: 11-24.
- H.T. Siegelmann, “Finite vs. Infinite Descriptive Length in Neural Networks and the Associated Computational Complexity,” in Finite vs. Infinite: Contributions to an Eternal Dilemma, C. Calude and G. Paun (eds.), Springer Verlag, 2000.
- H.T. Siegelmann, “Neural Automata and Computational Complexity,” in Handbook of Brain Theory and Neural Networks, M.A. Arbib (ed.), 2000.
- H. Lipson and H.T. Siegelmann, “High Order Eigentensors as Symbolic Rules in Competitive Learning,” in Lecture Notes in Computer Science 1778, Hybrid Neural Systems, Springer-Verlag, 1998: 286-297.
- H.T. Siegelmann, “Neural Dynamics with Stochasticity,” in Adaptive Processing of Sequences and Data Structures, C.L. Giles and M. Gori (eds.), Springer, 1998: 346-369.
- H.T. Siegelmann, “Computability with Neural Networks,” in Lectures in Applied Mathematics 32, J. Reneger, M. Shub, and S. Smale (eds.), American Mathematical Society, 1996: 733-747.
- H.T. Siegelmann, “Neural Automata,” in Shape, Structures and Pattern Recognition, D. Dori and F. Bruckstein (eds.), World Scientific, 1995:241-250.
- H.T. Siegelmann, “Towards a Neural Programming Language,” in Shape, Structures and Pattern Recognition, D. Dori and F. Bruckstein (eds.), World Scientific, 1995.
- H.T. Siegelmann, “Welcoming the Super-Turing theories,” in Lecture Notes in Computer Science 1012, M. Bartosek, J. Staudek, J. Wiedermann (eds.), Springer Verlag, 1995: 83-94.
- H.T. Siegelmann, “Recurrent Neural Networks,” in The 1000th Volume of Lecture Notes in Computer Science: Computer Science Today, J. Van Leeuwen (ed.), Springer Verlag, 1995: 29-45.
- H.T. Siegelmann, B.G. Horne, and C.L. Giles, “What NARX Networks Can Compute,” in Lecture Notes in Computer Science: Theory and Practice of Informatics Vol. 1012, M. Bartosek, J. Staudek, J. Wiedermann (eds.), Springer Verlag, 1995: 95-102.
- DasGupta, H.T. Siegelmann, and E. Sontag, “On the Intractability of Loading Neural Networks,” in Theoretical Advances in Neural Computation and Learning, V.P. Roychowdhury, K.Y. Siu, and A. Orlitsky (eds.), Kluwer Academic Publishers, 1994: 357-389.
- H.T. Siegelmann, “On the Computational Power of Probabilistic and Faulty Neural Networks,” in Lecture Notes in Computer Science 820: Automata, Languages and Programming, S. Abiteboul and E. Shamir (eds.), Springer Verlag, 1994: 20-34.
- H.T. Siegelmann and O. Frieder, “Document Allocation in Multiprocessor Information Retrieval Systems,” in Lecture Notes in Computer Science 759: Advanced Database Concepts and Research Issues, N.R. Adam and B. Bhargava (eds.), Springer Verlag, November 1993: 289-310.
- H.T. Siegelmann, E.D. Sontag, and C.L. Giles, “The Complexity of Language Recognition by Neural Networks,” Algorithms, Software, Architecture (J. Van Leeuwen, ed.), North Holland, Amsterdam, 1992: 329-335.
In Proceedings
- Gain, H. Siegelmann, “Abstraction Mechanisms Predict Generalization in Deep Neural Networks,” International conference on Machine learning (ICML), May 2020
- G. M. van de Ven, H. T. Siegelmann, A.S. Tolias “Brain-like replay for continual learning with artificial neural networks,” International Conference on Learning Representations (ICLR) workshop “Bridging AI and Cognitive Science,” April 2020. Selected for 15-min oral (6% acceptance rate). URL: https://baicsworkshop.github.io/program/baics_8.html
- A. Gain, P. Kaushik, H. Siegelmann, “Adaptive Neural Connections for Sparsity Learning,” The IEEE Winter Conference on Applications of Computer Vision (WACV), 2020, pp. 3188-3193
- A. Gain, H. Siegelmann, “Utilizing full neuronal states for adversarial robustness,” Proceedings Volume 11197, SPIE Future Sensing Technologies; 1119712 (2019), Tokyo, Japan https://doi.org/10.1117/12.2542804
- R. Kozma, R. Noack, H.T. Siegelmann (2019) Models of Situated Intelligence Inspired by the Energy Management of Brains, Proc. IEEE Inf. Conf. Systems, Man, and Cybernetics, SMC2019, October 5-9, 2019, Bari, Italy, IEEE Press.
- H. Hazan, D. Saunders, D. Sanghavi, H. T. Siegelmann and K. Robert, “Unsupervised Learning with Self-Organizing Spiking Neural Networks,” IEEE/INNS International Joint Conference on Neural Networks, Brazil, July 2018.
- D. Saunders, H. T. Siegelmann, R. Kozma and M. Ruszinko, “STDP Learning of Image Features with Spiking Neural Networks,” IEEE/INNS International Joint Conference on Neural Networks, Brazil, July 2018.
- R. Kozma, R. Ilin, and H. T. Siegelmann, “Evolution of Abstraction Across layers in deep learning neural networks,” INNS Big Data Deep Learning Conference (BDDL2018), Bali Indonesia, April 17-19 2018.
- R. Noack, C. Manjesh, M. Ruszinko, H. Siegelmann, and R. Kozma, “Resting State Neural Networks and Energy Metabolism,” IEEE/INNS International Joint Conference on Neural Networks, Anchorage Alaska, May 14-19 2017.
- J. Nick Hobbs and H.T. Siegelmann, “Implementation of Universal Computation via Small Recurrent Finite Precision Neural Networks” IEEE/INNS International Joint Conference on Neural Networks, Ireland, July 2015.
- A.S. Younger, E. Redd, H. Siegelmann “Development of Physical Super-Turing Hardware.” O.H. Ibarra et al. (Eds.) UCNC 2014 (Unconventional computation and Natural computation) Ontario, Canada, June, LNCS 8553 (2014): 379-391.
- A. Tal and H.T. Siegelmann, “Conscience mechanism in neocortical competitive learning,” ICCN2013 (International Conference on Cognitive Neurodynamics), Sigtuna, Sweden, June 2013.
- M. M. Olsen and H.T. Siegelmann, “Multiscale Agent-Based Model for Tumor Angiogenesis,” International Conference on Computational Science ICCS, June 2013: 1016-1025.
- J. Cabessa and H.T. Siegelmann, “Evolving Recurrent Neural Networks are Super-Turing,” Proceedings of International Joint Conference on Neural Networks; 2012 July 31 – August 5; San Jose, California, USA: 3200-3206.
- Harrington, K. I., M. Olsen, and H. Siegelmann, “Computational Neuroecology of Communicated Somatic Markers”. In Proceedings of Artificial Life XIII, July 2012: 555-556.
- K.I. Harrington, M.M. Olsen and H.T. Siegelmann, “Communicated Somatic Markers Benefit Both the Individual and the Species,“ Proceedings of International Joint Conference on Neural Networks; 2012 July 31 – August 5; San Jose, California, USA: 3272-3278.
- K. Tu, M. Olsen, H. Siegelmann. “CIM for Improved Language Understanding,” Proceedings of the Tenth International Symposium on Logical Formalization on Commonsense Reasoning. March 2011.
- Y. Z. Levy, D. Levy, J.S. Meyer, H.T. Siegelmann, “Identification and Control of Intrinsic Bias in a Multiscale Computational Model of Drug Addiction,” Proceedings of the 2010 Symposium on Applied Computing (ACM SAC 2010), Sierre, Switzerland, March 2010: 2389-2393.
- K. Tu and H.T. Siegelmann, “Text-based Reasoning with Symbolic Memory Model,” Proceedings of the Fifth International Workshop on Neural-Symbolic Learning and Reasoning (NeSy’09), Pasadena, USA, July 11, 2009: 16-21.
- M. Olsen, N. Siegelmann-Danieli, H. Siegelmann. Computational Modeling Reveals the Crucial Role of Cellular Citizenship in Selective Tumor Apoptosis. Systems Biology of Human Disease. June 2009.
- Y.Z. Levy, D. Levy, J.S. Meyer and H.T. Siegelmann, ”Drug Addiction: a computational multiscale model combining neuropsychology, cognition, and behavior,” Intl. Conf. on Bio-inspired Systems and Signal Processing (BIOSIGNALS), Portugal, 2009: 87-94.
- Y.Z. Levy, D. Levy, J.S. Meyer and H.T. Siegelmann, “Drug Addiction as a Non-monotonic Process: a Multiscale Computational Model,” 13th Intl. Conf. on Biomedical Engineering (ICBME), Singapore, December 2008. 4 pages.
- M. Olsen and H. Siegelmann, “Multi-Agent System that Attains Longevity via Death,” Proceedings of the Twentieth International Joint Conference on Artificial Intelligence (IJCAI), India, Jan 2007: 1428-1433.
- M. Olsen, H. Siegelmann. Artifical Death for Attaining System Longevity. Proceedings of the 50th Anniversary Summit of Artificial Intelligence Summit. pp. 217-218. July 2006.
- Y. Guo and H. Siegelmann, “Time-Warped Longest Common Subsequence Algorithm for Music Retrieval,” International Conference on Music Information Retrieval (ISMIR), Spain, October 2004: 258-261.
- T. Jaakkola and H. Siegelmann, “Active information retrieval,”
Advances in Neural Information Processing Systems (NIPS), Denver Colorado, 2001: 777-784. - P. Rodrigues, J. Félix Costa, H. T. Siegelmann, “Verifying Properties of Neural Networks,” International Work Conference on Artificial Neural Networks (IWANN), Granada Spain, June 2001: 158-165.
- D. Horn, I. Opher, M. Epstein and H. T. Siegelmann, ”Clustering of Documents using Latent Semantic Analysis,” Proceedings of the Document Analysis Systems (DAS), Rio de Janeiro, 2000.
- A. Ben-Hur, D. Horn, H.T. Siegelmann and V. Vapnik, “A Support Vector Method for Hierarchical Clustering,” Fourteenth Annual Conference on Neural Information Processing Systems (NIPS), Denver Colorado, 2001: 367-373.
- Ben-Hur, D. Horn, H.T. Siegelmann and V. Vapnik, “A Support Vector Clustering Method,” Proceedings of the 15th International Conference on Pattern Recognition (ICPR), Barcelona Spain, September 2000: 728-731.
- H.T. Siegelmann, A. Roitershtein and A. Ben-Hur, “Noisy Neural Networks and Generalizations,” Proceedings of Thirteenth Annual Conference on Neural Information Processing Systems (NIPS), Denver Colorado, December 1999: 335-341.
- H.T. Siegelmann and S. Fishman, “Attractor Systems and Analog Computation,” Proceedings of the Second International Conference on Knowledge-Based Intelligent Electronic Systems (KES’98), Adelaide Australia, 21-23 April 1998.
- H. Lipson, Y. Hod, and H.T. Siegelmann, “High-Order Clustering Metrics for Competitive Learning Neural Networks,” Proceedings of the Israel-Korea Bi-National Conference on New Themes in Computer Aided Geometric Modeling, Tel-Aviv Israel, February 1998: 181-188.
- J.P. Neto, H.T. Siegelmann, and J.F. Costa, “Turing Universality of Neural Nets Revisited,” Proceedings of the Sixth International Conference on Computer Aided Systems Technology (EUROCAST’97). In Franz Pichler and Roberto Moreno-Diaz (eds.), Lecture Notes in Computer Science (LNCS) 1333, 1997: 3651-366.
- D.H. Lange, H.T. Siegelmann, H. Pratt, and G.F. Inbar, “A Generic Approach for Identification of Event Related Brain Potentials via a Competitive Neural Network Structure,” Proceedings of the International Conference on Neural Information Proceeding (NIPS), Denver Colorado, December 1997: 901-907.
- Y. Finkelstein and H.T. Siegelmann, “A Stochastic Model to Study Degenerative Disorders in the Central Nervous System,” The Israel Neurological Association Annual Meeting, Zichron-Yaakov, November 1997.
- H.T. Siegelmann and S. Fishman, “Computation in Dynamical Systems,” Proceedings of the IEEE International Conference on Systems, Man, and Cybernetics, October 1997.
- H.T. Siegelmann, A. Ofri, and H. Guterman, “Applying Modular Networks and Fuzzy Logic Controllers to Nonlinear Flexible Structures,” Fuzzy Information Processing Society, Annual Meeting of the North American, September 1997: 96-101.
- G. Arieli and H.T. Siegelmann, “ANN Approach vs. the Symbolic Approach in AI,” Proceedings of the Thirteenth Israeli Conference on Artificial Intelligence and Computer Vision (IAICV’97), Tel-Aviv, February 1997.
- J. Utans, J. Moody, S. Rehfuss, and H. T. Siegelmann, “Selecting Input Variables via Sensitivity Analysis: Application to Predicting the U.S. Business Cycle,” Proceedings of Computational Intelligence in Financial Engineering, IEEE Press, New York, April 1995: 118-122.
- H.T. Siegelmann, “Recurrent Neural Networks and Finite Automata” Proceedings of the Twelfth International Conference on Pattern Recognition, Jerusalem, October 1994.
- E. Nissan, H.T. Siegelmann, and A. Galperin, “An Integrated Symbolic and Neural Network Architecture for Machine Learning in the Domain of Nuclear Engineering,” Proceedings of the Twelfth International Conference on Pattern Recognition, Jerusalem, October 1994: 494-496.
- E. Nissan, H.T. Siegelmann, A. Galperin, and S. Kimhi, “Towards Full Atomization of the Discovery of Heuristics in a Nuclear Engineering Project: Integration with a Neural Information Language,” Proceedings of the Eight International Symposium on Methodologies for Intelligent Systems, Charlotte, North Carolina, October 1994 (869): 427-436.
- H.T. Siegelmann, “Neural Programming Language,” Proceedings of the Twelfth National Conference on Artificial Intelligence, AAAI-94, July–August 1994, Seattle Washington, AAAI Press/The MIT Press, 1994, Vol. 2: 877-882.
- DasGupta, H.T. Siegelmann, and E. Sontag, “On a Learnability Question Associated to Neural Networks with Continuous Activations,” Proceedings of the Sixth ACM Workshop on Computational Learning (COLT), New Brunswick NJ, July 1994: 47-56.
- H.T. Siegelmann, “On the Computational Power of Probabilistic and Faulty Neural Networks,” Proceedings of the International Colloquium on Automata, Languages, and Programming (ICALP), Jerusalem, July 1994: 23-34.
- J. Kilian and H.T. Siegelmann, “Computability with the Classical Sigmoid,” Proceedings of the Fifth ACM Workshop on Computational Learning (COLT), Santa Cruz, July 1993: 137-143.
- H.T. Siegelmann and O. Frieder, “Document Allocation In Multiprocessor Information Retrieval Systems,” Advanced Database Systems, 1993: 289-310.
- H.T. Siegelmann and E.D. Sontag, “Analog Computation via Neural Networks,” Proceedings of the Second Israel Symposium on Theory of Computing and Systems (ISTCS), Natanya Israel, June 1993: 98-107.
- J.L. Balcázar, R. Gavalda, H.T. Siegelmann, and E.D. Sontag, “Some Structural Complexity Aspects of Neural Computation,” Proceedings of the IEEE Conference on Structure in Complexity Theory, San Diego, California, May 1993: 253-265.
- H.T. Siegelmann and E.D. Sontag, “Some Recent Results on Computing with ‘Neural Nets’,” Proceedings of the IEEE Conference on Decision and Control, Tucson Arizona, December 1992: 1476-1481. Best Student Paper Award.
- H.T. Siegelmann and E.D. Sontag, “On the Computational Power of Neural Networks,” Proceedings of the Fifth ACM Workshop on Computational Learning Theory (COLT), Pittsburgh Penn, July 1992: 440-449.
- H.T. Siegelmann and O. Frieder, “The Allocation of Documents in Multiprocessor Information Retrieval Systems: An Application of Genetic Algorithms,” Proceedings of the IEEE Conference on Systems, Man, and Cybernetics, Charlottesville Virginia, October 1991 (1): 645-650.
- O. Frieder and H.T. Siegelmann, “On the Allocation of Documents in Information Retrieval Systems,” Proceedings of the ACM Fourteenth Conference on Information Retrieval (SIGIR), Chicago Illinois, October 1991: 230-239.
- H.T. Siegelmann and B.R. Badrinath, “Integrating Implicit Answers with Object-Oriented Queries,” Proceedings of the Conference on Very Large Data Bases, Barcelona Spain, September 1991: 15-24.
Abstracts and Short Papers
- A. Gain and H. Siegelmann, Relating information complexity and training in deep neural networks,” SPIE Defense + Commercial Sensing, 2019, Baltimore, Maryland, United States
- K. Tu, H. T. Siegelmann, “Memory Model for Text Reasoning,” Northeast Student Conference on Artificial Intelligence (NESCAI) 2010.
- M. Olsen, R. Sitaraman, N. Siegelmann-Danieli, H. Siegelmann, “Mathematical and computational models for cellular space in cancer growth,” Proceedings of the American Association for Cancer Research. April 2010.
- M. M. Olsen, N. Siegelmann-Danieli and H.T. Siegelmann, “Mathematical and computational models for cellular space in cancer growth,” American Association for Cancer Research (AACR) 101th Annual meeting, Washington D.C., April 2010.
- Y.Z. Levy, D. Levy, J.S. Meyer, H.T. Siegelmann (2009). “Ceasing the use of narcotics without treatments in the context of a multiscale computational model of addiction,” 6th annual meeting of the Society for Autonomous Neurodynamics, Principles of Autonomous Neurodynamics 2009 (SAND), La Jolla, CA, USA, July 2009.
- Y.Z. Levy, D. Levy, J.S. Meyer, H.T. Siegelmann. Neuropsychology, cognition, and behavior of drug addiction: A non-monotonic multiscale computational model. 13th International Conference on Cognitive and Neural Systems (ICCNS), Boston, MA, USA, May 2009.
- D. Nowicki and H.T. Siegelmann, “The Secret Life of Kernels: Reconsolidation in Flexible memories,” Computational and Systems Neuroscience (COSYNE), February 2009. doi: 10.3389/conf.neuro.06.2009.03.271
- H.T. Siegelmann, M. M. Olsen and N. Siegelmann-Danieli, “Rescue Selective Apoptosis Relies on Cell Communication and Citizenship Commitments: A Computational Approach,” American Association for Cancer Research (AACR) 99th Annual Meeting, Dan Diego, April 2008.
- M. M. Olsen, K. Harrington and H.T. Siegelmann, “Emotions for Strategic Real-Time Systems,” AAAI Spring Symposium on Emotion, Personality and Social Behavior, Technical Report (SS-08-04), March 2008: 104-110.
- D. G. Cooper, D. Katz and H.T. Siegelmann, “Emotional Robotics: Tug of War,” AAAI Spring Symposium on Emotion, Personality and Social Behavior, Technical Report (SS-08-04), March 2008: 23-29.
- L. E. Holtzman and H.T. Siegelmann, “Input driven dynamic attractors,” Computational and Systems Neuroscience (COSYNE), Salt Lake City, February 2007: 101.
- M. M. Olsen, H.T. Siegelmann, “Artificial Death for Attaining System Longevity,” Proceedings of the 50th Anniversary Summit of Artificial Intelligence, Switzerland, July 2006: 217-218.
- K. Harrington and H.T. Siegelmann “Adaptive Multi-Modal Sensors,” Proceedings of the 50th Anniversary Summit of Artificial Intelligence, Switzerland, July 2006: 163-164.
- W. Bush and H.T. Siegelmann, “Genetic based neurons,” Computational and Systems Neuroscience (COSYNE), Salt Lake City, 2005: 69.
- E. Bittman, Y. Chait, C.V. Hollot, M. Harrington and H. Siegelmann, “Is the Mammalian Circadian Clock a Resonant-Circuit Oscillator?” Society for Research on Biological Rhythms, Whistler, BC, 2004.
- Y. Tong and H. Siegelmann, “Simulation mammalian molecular circadian oscillators by dynamic gene network,” Eighth Annual International Conference on Research in Computational Molecular Biology (RECOMB), San Diego CA, March 2004.
- S. Lu, A. Guo, K. Becker, H. Siegelmann, P. Sebastiani, K. MacBeth, J. Jerry, “Microarray Analysis of Global Gene Expression in the Mammary Gland Following Estrogen and Progesterone Treatment of Ovariectomized Mice,” Second Annual AACR International Conference on Frontiers in Cancer Prevention Research, Phoenix, Arizona, October 2003.